Welcome to HPI Knowledge - the publications platform of the Hasso Plattner Institute! The platform provides quick access to our publications. Explore recent papers and key insights from fundamental research to real-world applications which shape the digital future.
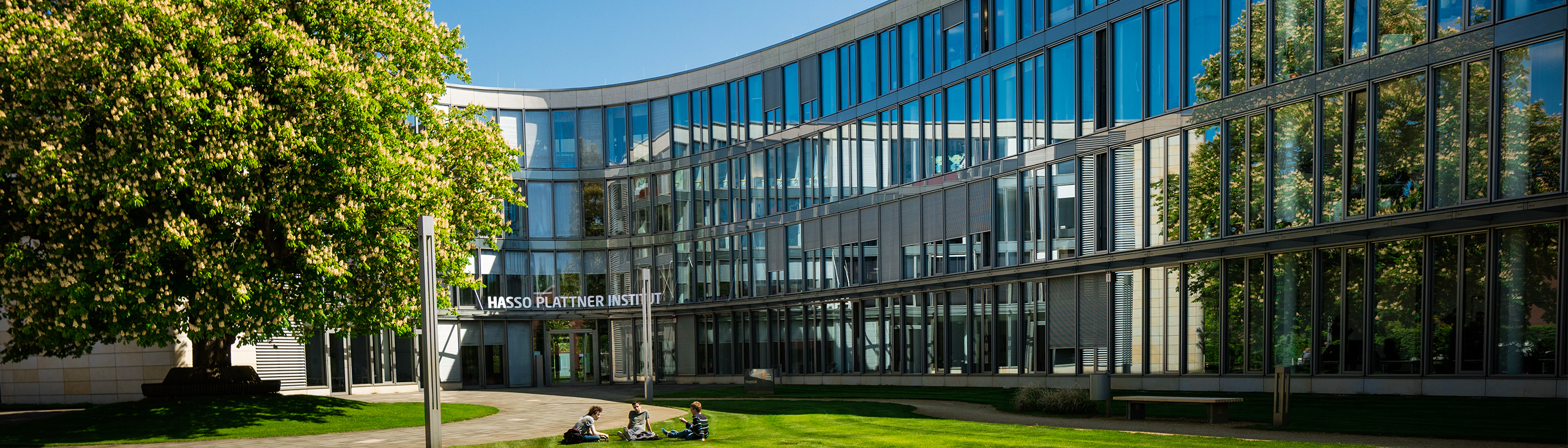
Recent Additions
- Some of the metrics are blocked by yourconsent settings
Publication
Most viewed
- Some of the metrics are blocked by yourconsent settings
Publication - Some of the metrics are blocked by yourconsent settings
Publication Runtime Analysis for Multi-Objective Evolutionary Algorithms in Unbounded Integer Spaces(2025)Randomized search heuristics have been applied successfully to a plethora of problems. This success is complemented by a large body of theoretical results. Unfortunately, the vast majority of these results regard problems with binary or continuous decision variables -- the theoretical analysis of randomized search heuristics for unbounded integer domains is almost nonexistent. To resolve this shortcoming, we start the runtime analysis of multi-objective evolutionary algorithms, which are among the most successful randomized search heuristics, for unbounded integer search spaces. We analyze single- and full-dimensional mutation operators with three different mutation strengths, namely changes by plus/minus one (unit strength), random changes following a law with exponential tails, and random changes following a power-law. The performance guarantees we prove on a recently proposed natural benchmark problem suggest that unit mutation strengths can be slow when the initial solutions are far from the Pareto front. When setting the expected change right (depending on the benchmark parameter and the distance of the initial solutions), the mutation strength with exponential tails yields the best runtime guarantees in our results -- however, with a wrong choice of this expectation, the performance guarantees quickly become highly uninteresting. With power-law mutation, which is an essentially parameter-less mutation operator, we obtain good results uniformly over all problem parameters and starting points. We complement our mathematical findings with experimental results that suggest that our bounds are not always tight. Most prominently, our experiments indicate that power-law mutation outperforms the one with exponential tails even when the latter uses a near-optimal parametrization. Hence, we suggest to favor power-law mutation for unknown problems in integer spaces. - Some of the metrics are blocked by yourconsent settings
Publication Functional Dependency Discovery: An Experimental Evaluation of Seven Algorithms(VLDB Endowment, 2015)Functional dependencies are important metadata used for schema normalization, data cleansing and many other tasks. The efficient discovery of functional dependencies in tables is a well-known challenge in database research and has seen several approaches. Because no comprehensive comparison between these algorithms exist at the time, it is hard to choose the best algorithm for a given dataset. In this experimental paper, we describe, evaluate, and compare the seven most cited and most important algorithms, all solving this same problem. First, we classify the algorithms into three different categories, explaining their commonalities. We then describe all algorithms with their main ideas. The descriptions provide additional details where the original papers were ambiguous or incomplete. Our evaluation of careful re-implementations of all algorithms spans a broad test space including synthetic and real-world data. We show that all functional dependency algorithms optimize for certain data characteristics and provide hints on when to choose which algorithm. In summary, however, all current approaches scale surprisingly poorly, showing potential for future research. - Some of the metrics are blocked by yourconsent settings
Publication